Use cases
Columbus
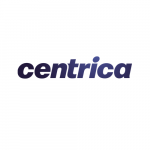
How Columbus helped a multinational electricity company reduce customer churn
illumr identified that Centrica customers who made fewer calls were more likely to cancel their contracts.
Using Columbus, the company can now target these customers, persuade them to stay and reduce churn.
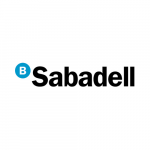
How Columbus helped a European bank get greater customer value
illumr identified the key drivers determining which customers of Sabadell Bank would increase their value in the future.
The bank can now target these customers effectively for increased revenue.
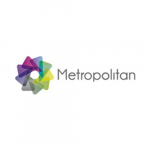
How Columbus helped a housing association fight domestic abuse
Metropolitan housing association was trying to identify households where tenants were more likely to be the subject of a safeguarding incident. But AI had failed to reveal any insights.
Columbus uncovered unique insights from the sparse dataset that no other methodologies could find.
We identified four key clusters of homes where tenants were 10-50x more likely to be at risk of a safeguarding incident.
Metropolitan can now decisively act to help safeguard tenants against potential risks.
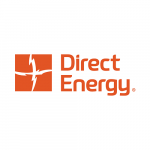
How Columbus helped a US energy company reduce customer churn
Columbus identified Direct Energy’s long-term gas customers, with good margins. We found that these customers were likely to leave Direct Energy when they encountered issues.
The energy provider can now better target these customers to improve retention rates and reduce churn.
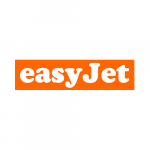
How Columbus helped easyjet communicate effectively with customers
The challenge
Analytical methods had failed to identify natural segments of customers within easyJet’s data.
Segments are often chosen apriori (e.g. holidaymakers, businesspeople). Customers are then forced to fit into one of those categories. The airline’s inability to accurately segment customers meant their targeted marketing was ineffective.
The solution
A cluster was chosen and analysed – it found customers could offer 8x more value for easyJet on average.
In the identified cluster, customers travelled for both business and leisure purposes. Forcing customers into one or the other therefore would have failed to identify this high value group.
The result
The airline is now better able to understand its customers’ behaviours. It can identify natural segments of customers free of human bias.
easyJet can now effectively tailor its marketing for increased sales and customer engagement.
Rosa
How Rosa found and lowered racial bias from a criminal risk assessment tool
The challenge
The Correctional Offender Management Profiling for Alternative Sanctions (COMPAS) is a 137 question assessment. Its purpose is to determine the likelihood that a convict will reoffend.
It produces a risk score between 1 and 10 - a score of 10 is the highest risk possible. However, there is no guidance on how this translates into actual likelihood of reoffending.
An indicator of bias is the difference in the ratio between False Negative Rates and False Positive Rates for black and white defendants. Even though the overall predictive accuracy is similar for different races, the algorithm makes mistakes in different ways, depending on the race of the defendant.
The solution
COMPAS tends to overestimate the likelihood of reoffending for black defendants. It also underestimates the likelihood of white defendants reoffending. This is clear evidence that the algorithm is biased with respect to race.
illumr was set the same task as COMPAS - to predict reoffending. We used Rosa to de-bias the data.
The result
While all tests suggested significant racial bias, the bias in the Rosa model had a 30x lower effect size than COMPAS. Its predictions were also more accurate.
It is possible to remove bias without seriously compromising predictive performance, avoiding the pitfalls of more common methodologies.